Project AppMOOC
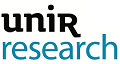
The project AppMOOC (Support for semi-automatic correction of online activities in massive courses (MOOCs)) is designing and implementing a software support for semi-automatic correction of activities in massive courses (> 200 students per course). In this way, the teacher may receive a pre-assessment of each student, grouped by profiles and results, which will benefit the correction process (in time and depth), learning, and allow greater and more adequate feedback to the student.
The aim is that this system can work in a transparent and coordinated manner with the content management system used by the academic institution. Combining academic monitoring with ongoing evaluation activities, such as forums, sessions, wikis, etc., and formal summative evaluation activities such as tests and oral defenses.
Thus, the teacher / tutor can make a personalized monitoring of students, and get a detailed report of their activity, including an identification of their pattern of behavior (social activity) and performance (academic activity), which allow pre- evaluate your itinerary. AppMOOC do not replace the correction process, but facilitates to the teacher the pre-categorization of student outcomes. Thus, the process is streamlined, creating two possible benefits, not mutually exclusive:
- The process is shortened, allowing the teacher to focus more on individualized attention to students, because he has more time mentoring for it;
- The process is parameterized, allowing the teacher to take on more students, and that mass open courses (MOOC) have an effective mechanism of interaction with a real teacher; This approach allows interactivity with an expert, not just a set of automated tests without human intervention, as usually happens.
Innovations and Advantages of the project:
The first innovative element is the conceptual framework for semi-automatic correction mass activities courses itself. The recommendation algorithms and processes are typically based on two principles: collaboration between users (collaborative filter) and available platform content (content-based). In response to literature, both approaches have proved to be quite efficient, and much less when generating recommendations in the field of eLearning. There are other more complex approaches that try to fill gaps in the line just mentioned but have not gone through the necessary validation of eLearning production environments.
AppMOOC, as a conceptual framework rests on the initial supervision of an expert (teacher, tutor or a member of the teaching community) who is in charge of weighing and balancing the contribution of the different categories of data collected based on course objectives, group or field of study. Likewise, the conceptual framework nor suffer the obstacle called cold-start itself of recommender systems based on collaborative filtering, but, since the beginning, AppMOOC is already trained to operate. These features make suggestions oriented to educational practical and integrated teaching consistently with each particular environment pattern.
The second innovation is the technical implementation itself: AppMOOC. As software and computer system AppMOOC behaves as middleware between a manager learning (LMS) and the user (teacher, tutor, student) so that it is able to generate eLearning recommendations regardless of the system used. This requires use of LTI and schedule a simple standard APIs that allow connection to realize integration with leading LMS (i.e. Sakai, Moodle, Blackboard, etc.). On the other hand, the interface configuration has been designed from the beginning to present a very intuitive, clean and ready to use by the expert. It has placed particular emphasis on the use of open-platform technologies such as HTML5. This approach will allow the use of AppMOOC in tablets, smartphones and other devices overall performance.
Call ADER 2014 performance 1 “Conducting research and development projects”